Biologist, Data Analyst and Growth Hacker walk into a bar
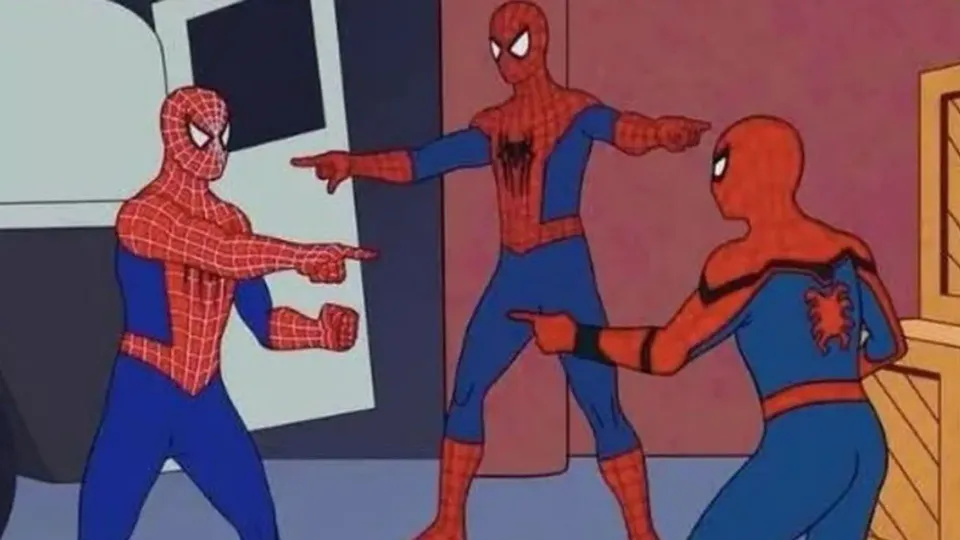
5 Growth Experimentation lessons I've learnt while working in SaaS
TL;DR:
Successful product growth has a strong reliance on the application of scientific rigor. Below are 5 key lessons that are derived from this cross-domain thinking.
- Ensure reliable outcomes through replicable and rigorous experiments with controlled processes. Design experiments so that you can attribute results directly to the independent variable.
- Embrace hypothesising to drive rational and evidence-based strategies. Specify key metrics and predicted outcomes in your hypotheses.
- Adopt iterative evolution by continuously drawing new hypotheses. Maximise product growth through incremental experiments.
- Rely on data rather than intuition. Conduct preliminary research and use data to validate experiment designs.
- Don't fear failure; use it as a learning opportunity. Keep a learning log and leverage lessons from failed experiments for future ideation.
Introduction
Product innovation and growth has a strong reliance on the successful application of scientific thinking. Speaking from experience, if you're a multifaceted individual with a technical background and curiosity for the product domain, this is good news.
From studying biology, to analysing and advising government policy, to data analytics at AgTech and FinTech startups, I've learnt to practice and leverage interdisciplinarity. This principle has helped me sprint into every challenge, including marketing and product experimentation in SaaS, and consciously leverage the unique and valuable experiences from seemingly different domains.
The biggest lesson to take away, and one that is obvious but needs to be reinforced, is that if we want to deliver success in any domain, we can achieve this by creating a framework that combines the effective principles and mindsets of other disciplines.
Biologist | Data Analyst | Growth Hacker | |
Mindset | Curious and observant. | Analytical storyteller. | Experimental, rapid and innovative. |
1. Replication and Rigor - Ensuring Reliable Outcomes
Poorly designed experiments can't be mitigated by data or good ideas.
Experiments need to be replicable and rigorous and must follow controlled processes, allowing for smooth evaluation of results. Using scientific rigor in product experimentation boosts confidence in decision-making by reducing uncertainties and establishing solid foundations for growth initiatives.
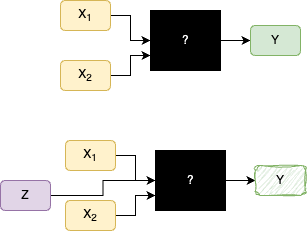
Lesson 1: For every iteration, limit experiments to the least number of changes per variant and keep all other variables fixed.
Mistake: You've implemented a new ad campaign to attract more users to the site but also made changes to the landing page. It has resulted in higher CTR but lower CvR compared to the control variant. We don't know with confidence what experimental change has caused these results.
Solution: The results should always be directly attributed to the independent variable. Validate the performance of a new ad campaign first while keeping landing page variables fixed or optimise the landing page CvR first. Always follow this framework of singular, iterative changes, even if we want to conclude how combinations of changes work together.
Lesson 2: Ensure to record the sample size of each variant and calculate the confidence level of each result when analysing the data.
Mistake: You stopped the analysis of results at aggregates without establishing whether the sample sizes are high enough to be confident in the results per variant. You implement the experiment with a larger sample size and see different results.
Solution: You can install the Stats Machina add-on for Google Sheets and use the =AB_CONFIDENCE() function as instructed. You can feel more confident in the likelihood that the results of the experiment are the closest to the truth at the time the experiment was run.
2. Embracing the Power of Hypotheses
Extending from the point above, hypothesising correctly ensures that every step towards growth is rooted in rational and evidence-based strategies.
Just as biologists formulate hypotheses to explain natural phenomena, entrepreneurs, marketers and product developers can adopt a hypothesis-driven mindset to achieve success. Hypothesising correctly ensures the team stays focused and is clear on the aims. It also provides relevant stakeholders with more clarity on the aim and outcome of the experiment, before implementing a positive change.
Lesson:
- Ask yourself if your hypothesis can be tested, and is based on a target (dependent variable) and something you change (independent variable).
- Include in your hypothesis 1) the relevant variables, 2) the specific group being studied, 3) the predicted outcome of the experiment or analysis.
Mistake: You haven't specified the key metrics in your hypothesis or the predicted change and impact. Improve this by specifying both, e.g. "Users are more likely to click on our signup button if we change the colour of our signup button from red to grey".
Solution: Follow a trusted hypothesis setting framework during every experiment development session. Here's a website that can walk you through the process.
3. Iterative Evolution
Avoid assuming an experiment is over.
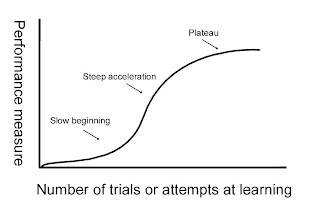
Experimentation in the sciences doesn't halt randomly. With each set of conclusions added to the domain knowledge pool, further hypotheses can be drawn. The same can be said within growth experimentation. Successful products persevere through iterative experiments, maximising their chances of growth and market dominance.
Lesson: Evaluate the potential return on investing in another iteration of the variable and use reduction in marginal changes in improvements to close an experiment off.
Mistake: You stopped at the first sign of success and not questioning whether similar variables could be adjusted, if not the same variable, but to different degrees. E.g., changing the text size on your landing page and concluding the experiment at a x% increase in conversion rate without considering other text formatting variables.
Solution: Based on the level of risk (determined nicely by the ICE method), start with a product variant and compare results through each iteration. When your % change in click-through rate, conversion rate or whichever metrics start to drop off, you've likely hit a growth plateau (this also correlate with your learning plateau) - you have juiced the experiment of its total value and can move on.
4. Data, Not Vibes:
Your intuition doesn't complete the picture, use data to confirm it.
Anecdotal insights help with the ideation process, but it shouldn't be the sole reason behind a change in a variable, especially if the risk of outcome is high. The optimal approach lies between intuition and data-driven insights.
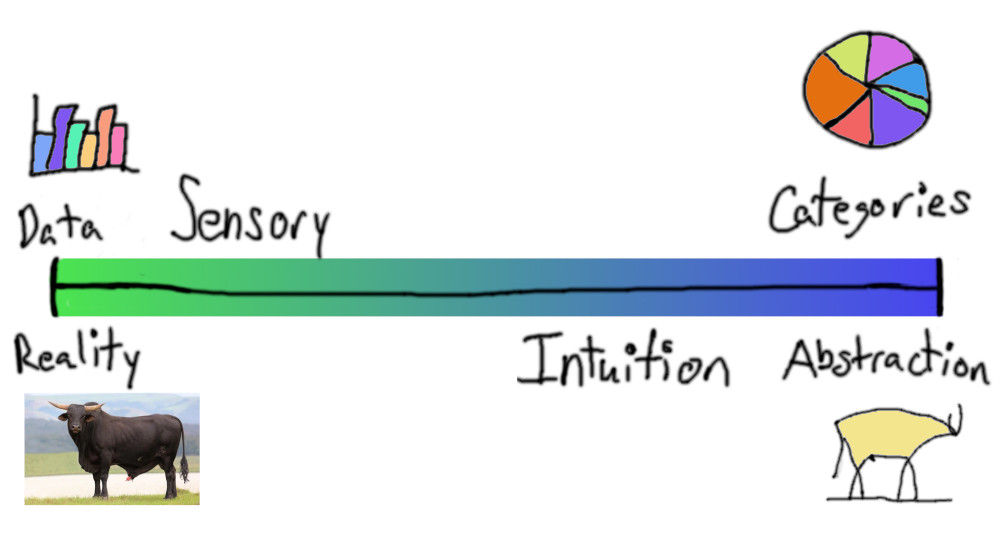
Lesson: Build on knowledge of customers gained from previous experiments or conduct preliminary research to test the premise of the hypothesis/experiment design.
Mistakes: You're testing the impact of new communication styles on retention without analysing engagement behaviour beforehand to validate which mode of communication is best e.g., if users don't login often, emails could be a more reliable way to test the impact of the messaging/content.
Solution: In this case, compare data on customer login recency and frequency, as well as email open rates, to determine the more effective platform for testing communication.
5. Fear of failure:
Don't shy away from results that make you sad. This one is pretty simple, but worth noting.
Lesson: The aim of experimentation is to answer questions, of which can be used to guide the direction of new questions.
Mistake: Abandoning the experiment because the click-through rate wasn't higher for the variant.
Solution: Create a learning log (a simple spreadsheet will do) of the lessons learned per iteration of an experiment. Leverage these learnings during experimentation ideation. This will help reframe the important of failed variants.
Thanks for reading!